Battery Management Systems
What’s a Battery Management System?
A Battery Management System (BMS) is an electronic system responsible for monitoring and controlling the operation of batteries. It performs a variety of functions to ensure the correct and safe functioning of batteries in various applications.
The BMS consists of microcontroller-based hardware and embedded software that work together to carry out its tasks. It utilizes advanced techniques such as State of X (SoX) estimation, where X represents different parameters such as State of Charge (SoC), State of Health (SoH), State of Energy (SoE), State of Power (SoP), and Remaining Useful Life (RUL).
The SoC estimation is crucial in determining the remaining capacity or energy stored in a battery. By monitoring the voltage, current, and other relevant parameters, the BMS can estimate the SoC, providing information about the available energy and the battery’s discharge state.
The SoH estimation focuses on assessing the health or condition of the battery. It takes into account factors such as capacity degradation, internal resistance, and other performance indicators. The BMS uses advanced algorithms to analyze these parameters and provide an estimation of the battery’s health, allowing for proactive maintenance and replacement strategies.
The SoE estimation relates to the available energy in the battery at a given time. It considers the current operating conditions, load requirements, and the battery’s internal characteristics to estimate the energy that can be delivered by the battery.
The SoP estimation deals with the power capability of the battery. It determines the maximum power that the battery can deliver under specific conditions. By monitoring parameters such as voltage sag, internal resistance, and temperature, the BMS assesses the battery’s ability to provide the required power for various applications.
RUL estimation is another essential aspect of the BMS. It involves predicting the remaining useful life of the battery. By analyzing historical data, usage patterns, and degradation characteristics, the BMS can estimate how much longer the battery will continue to meet performance requirements before it needs replacement or maintenance.
The integration of these functionalities within the BMS enables efficient monitoring, control, and optimization of battery performance. It ensures safe operation, extends battery lifespan, and maximizes the utilization of battery resources in applications ranging from consumer electronics to electric vehicles, renewable energy systems, and more.
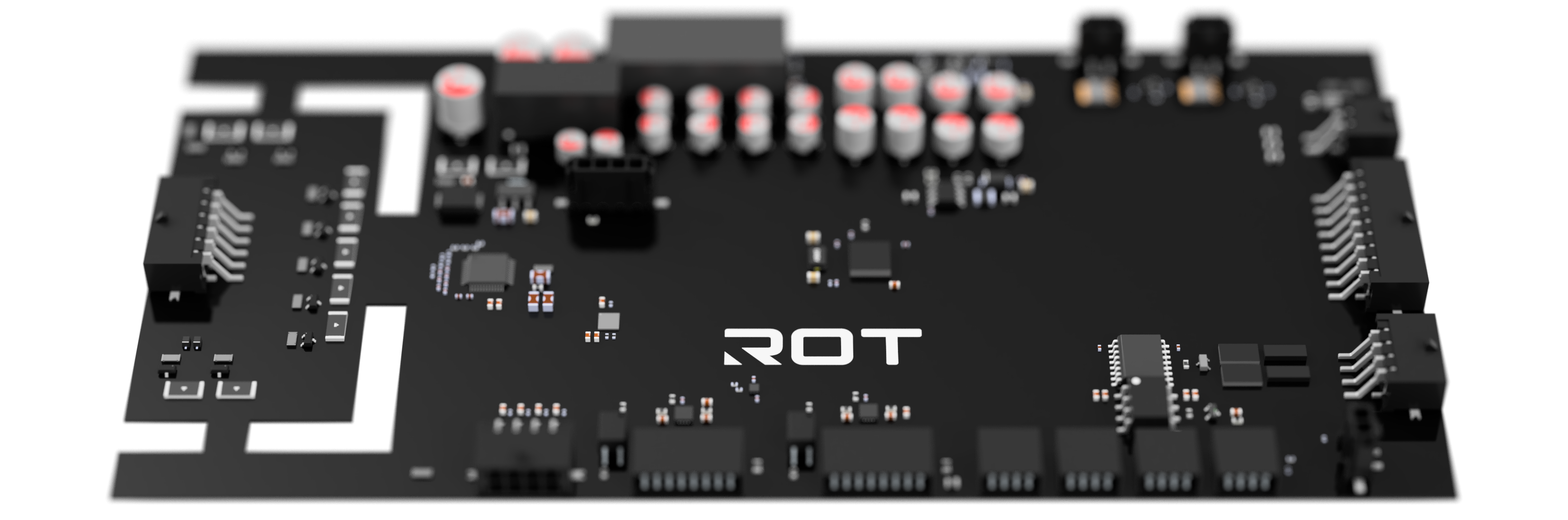
How does AI revolutionize BMS?
The integration of AI (Artificial Intelligence) and machine learning techniques has revolutionized the capabilities of Battery Management Systems (BMS). By harnessing the power of AI, the BMS can enhance its monitoring, control, and predictive analysis functions for batteries.
Through AI and machine learning, the BMS can analyze large volumes of data collected from batteries, enabling more accurate estimations and predictions regarding battery performance and health. These technologies enable the BMS to learn patterns, detect anomalies, and make intelligent decisions based on the data it gathers.
AI algorithms and machine learning techniques facilitate data analysis and pattern recognition within the BMS. By examining historical and real-time battery data, the BMS can identify patterns and correlations, detect trends in battery behavior, and predict future performance. This allows for proactive maintenance and interventions to address potential issues before they lead to failures.
Anomaly detection and fault diagnosis are also improved through AI-based BMS. By comparing real-time data with historical data, the BMS can identify abnormal behavior or deviations from expected battery performance. This enables the BMS to detect faults or potential failures in the battery system and take appropriate actions.
AI empowers the BMS to adaptively control and optimize battery operations. Machine learning algorithms can optimize charging and discharging profiles based on factors such as battery chemistry, temperature, and load requirements. This optimization maximizes battery life, performance, and efficiency.
Predictive maintenance and Remaining Useful Life (RUL) estimation are also enhanced through AI and machine learning. By analyzing battery data and usage patterns, the BMS can estimate the RUL of batteries. This enables proactive scheduling of maintenance activities, reducing the risk of unexpected failures and optimizing battery utilization.
Additionally, AI-based BMS can adapt to different battery chemistries and configurations. Machine learning algorithms can learn from data collected from various types of batteries and optimize their performance accordingly. This flexibility allows the BMS to be used across a wide range of applications and battery technologies.
By integrating AI and machine learning into BMS technology, battery monitoring, control, and decision-making processes are significantly improved. The accuracy of battery condition estimation is enhanced, enabling predictive maintenance strategies and optimizing battery performance and lifespan. These advancements contribute to the overall reliability, efficiency, and safety of battery-powered systems.